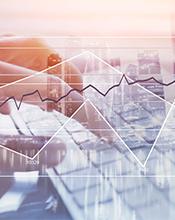
Lingfeng Lyu
Abstract: This paper presents a tri-level hierarchical approach to house price modelling at the postcode level, which is considered the most granular geographical scale, incorporating macroeconomic influences from the national level and integrating data from the largest sub-state level (SA4). By employing a Risk Premium - Principal Component Analysis (RP-PCA) for SA4-level risk factors and combining these with national-level risk factors, a vector autoregressive (VAR) model is developed. This geographically conditional multi-factor model with a hierarchical structure offers enhanced short-term prediction accuracy while maintaining long-term forecasting capabilities. The model’s predictive accuracy is further enhanced by introducing an empirical copula to describe the dependence structure of one-step residuals across various suburbs. This methodology grants a dynamic and granular view of housing price trends in Australia. Key determinants like interest rate shifts, GDP growth, and exchange rate variances play a crucial role, particularly in urban areas in metropolitan cities. The analysis of economic and demographic factors on the SA4 level indicates that elements such as home debt increments, wage fluctuations, and population shifts are pivotal in shaping housing prices, underscoring the significance of a granular regional analysis.
Keywords: House price modelling, Hierarchical framework, Macroeconomic variables, Risk Premium - Principal Component Analysis (RP-PCA)
